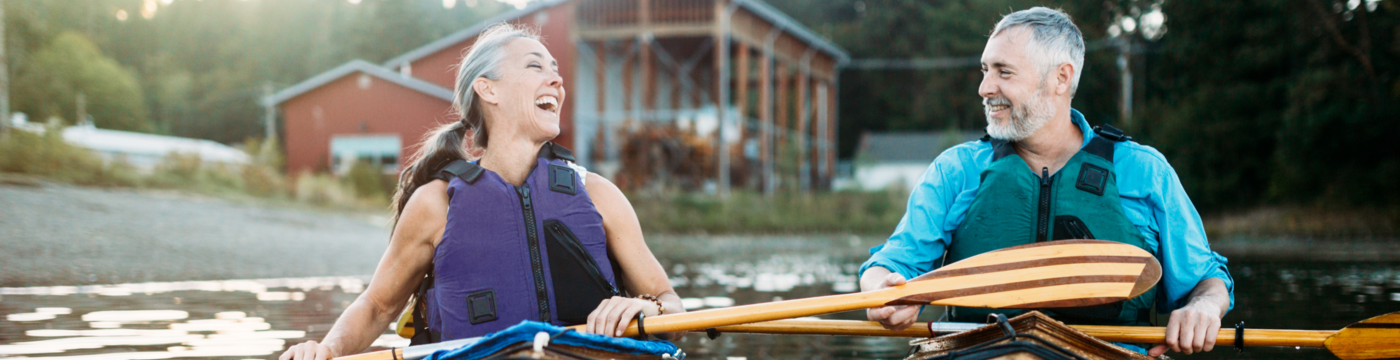
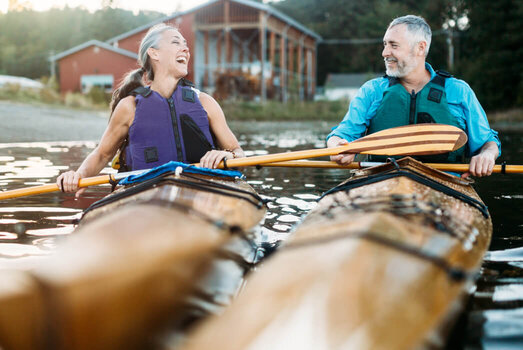
How it works
Predicting progression of Barrett's esophagus
TissueCypher was developed to overcome the challenges associated with risk-stratifying non-dysplastic Barrett's esophagus (NDBE) patients. The test characterizes molecular changes in Barrett's esophagus (BE) tissue that precede dysplasia, enabling the identification of progressors and nonprogressors at a treatable precancerous stage. The test uses biomarkers, spatial biology, and an AI-driven risk classifier to identify a patient’s five-year risk of progression to high-grade dysplasia (HGD) or esophageal adenocarcinoma (EAC).
Benefits of a spatialomics approach
Tumors are composed of many interacting cell types – including malignant cells; immune cells, such as macrophages; stromal cells, such as fibroblasts; and endothelial cells – as well as cancer stem cells. Spatialomics evaluates protein expression and the location where the expression occurs (spatial biology), providing a more complete picture of the molecular changes occurring in the BE tissue.

TissueCypher testing process
- Tissue blocks or slides from standard tissue biopsies are sent to our lab for testing
- Slides undergo multiplexed immunofluorescence staining to visualize nine biomarkers
- Stained slides are digitally scanned and 15 features are characterized by AI-driven image analysis
- The 15 features are characterized by AI-driven image analysis into a risk score from 0-10, a risk class of low, intermediate, or high-risk, and an associated 5-year risk of progression to HGD or EAC
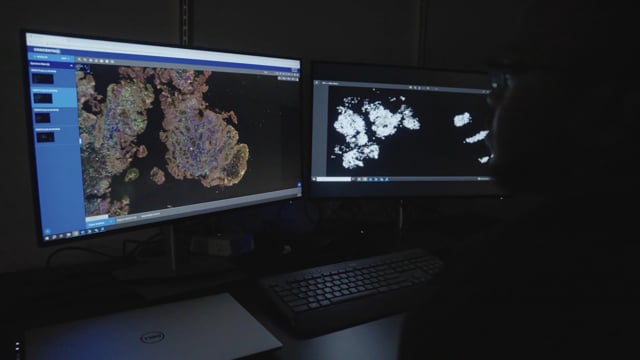
Interpreting the molecular signs of progression
TissueCypher uses immunofluorescence to evaluate the expression levels of nine protein biomarkers that are associated with cancer progression.
Protein Markers | Tissue Cypher Features |
p53, p16, AMACR | Loss of tumor suppression and cell cycle control |
CD68, COX2 | Immune and inflammatory markers |
HER-2, CK20 | Cancer growth and cell transformation |
HIF1alpha, CD45RO | Angiogenesis and memory lymphocyte infiltration |
TissueCypher’s vision system characterizes seven tissue structures to identify features that are used by the TissueCypher Risk Classifier.
TissueCypher evaluates 15 features that were shown during the GAPP1 Study to contribute to the predictive power of TissueCypher’s risk classifier.
Protein markers | Protein function |
p53 | p53 nuclear sum intensity |
p53 | p53 nuclear mean intensity |
HER2/neu and K20 | Ratio of mean HER2/neu intensity:mean K20 intensity in nuclei clusters |
HER2/neu and K20 | Ratio of 95th quantile HER2/neu intensity:95th quantile K20 intensity in nuclei clusters |
COX-2 and CD68 | Coexpression cellular COX2 mean intensity and cellular CD68, mean intensity |
p53 | p53 mean intensity in nuclei clusters |
p53, p16 and nuclear morphology (solidity) | Nuclear solidity in p53+ p16- cells |
CD45RO | CD45RO plasma membrane sum intensity |
AMACR | AMACR microenvironment SD |
COX2 | COX-2 texture in cytoplasm |
HIF1α | HIF1α microenvironment cell mean intensity |
HIF1α | HIF1α microenvironment cell moment (product of mean and standard deviation) |
p16 | p16 cytoplasm mean intensity |
p53, p16 and nuclear morphology (area) | Nuclear area in p53+ p16- cells |
Nuclear morphology | Hoechst nuclear 95th quantile intensity |